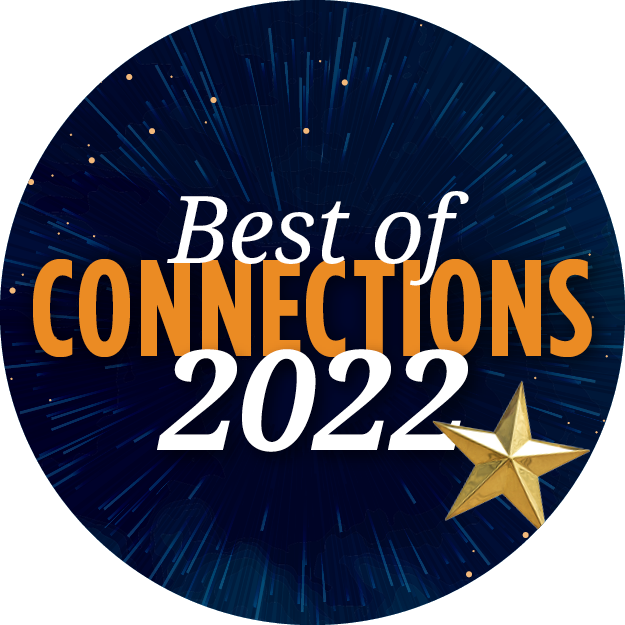
Editor's Note: This article is featured in Best of Connections 2022. Read the editor's message to learn more about the top articles of the year.
Fundraising is changing, due in part to an increasing focus on the impact of data governance within our organizations. The implications of this change for prospect researchers is likely to send ripple effects throughout the field, one of which is an increased responsibility in how organizations handle their data.
In parallel to this development, the field continues to break ground in implementing diversity, equity and inclusion (DEI) initiatives within organizations and philanthropy overall. As the fundraising profession slowly starts to adopt substantive policies toward fair and equitable philanthropy, prospect researchers will likely also serve as a conduit to implement data governance protocols within DEI initiatives (for example, see the Apra DEI Data Guide).
However, current conceptualizations of data governance are ill-equipped to substantively achieve the goals of DEI initiatives. Where data governance can be summed up as the implementation of rules, protocols and guidelines of how data is handled within organizations, it is mainly a project of organizational efficiency.
DEI within philanthropy, on the other hand, is a restorative project. It is about making identities whole through institutionalizing fair and equitable practices within our organizations — not just for our colleagues, but for the beneficiaries and constituents we serve. However, restorative projects by their very nature are not efficient. They are complicated processes without clear guidelines; to be successful they need unbounded spaces to see their purpose through.
DEI initiatives within philanthropy are such processes. If there will be a push in implementing data governance protocols in service of promoting DEI principles, our current understanding of data governance may hamper that process. The purpose of data governance is conceptualized as a project of minimizing risk and maximizing the efficiency of data flows within our organizations.
This does not mean data governance is unable to be just, equitable and fair. It does mean our conventional ways of understanding data governance do not go far enough in institutionalizing what is needed for DEI initiatives to truly be successful, and must evolve.
This transformation is possible. While there may be misalignment between data governance projects and DEI, there is common ground to align the purposes of each project. This article should not be seen as a how-to guide on the exact steps toward that goal. It is simply another voice in a conversation about DEI in our field, and how to stay true to its mission.
Data Consistency
When utilizing data governance in service of DEI initiatives, an either-or choice between organizational efficiency and being equitable is not necessary. Both can and must exist simultaneously if DEI initiatives are to be integral to how an organization runs its business.
By unpacking how data governance and DEI are misaligned, we can identify common ground between projects for a more suitable alignment. Finding such overlaps allows us to engage with data governance as a project of efficiency, and evolve that project to take into effect the necessary complexity of restorative projects like DEI.
One such overlap is the responsibility of data governance to ensure the consistency of how data is understood within an organization. In the traditional context, this might mean implementing rules and protocols for labeling data, establishing a metadata structure, mapping of database tables, etc. From an efficiency standpoint, the conventional goal of data governance in this dynamic — ensuring that everyone’s understanding of the data is consistent — allows that information to be coherent, actionable and fully leveraged toward the organization’s goals. When these conventions are simply implemented in efforts for efficiency, however, we are likely to make sacrifices within DEI initiatives as well.
To avoid this outcome, we should instead establish a common goal between data governance and DEI principles: ensuring that meaning is consistent within our organizational data. For example, the definitions of a prospect, donor or major gift must be consistently defined in all organizational contexts, in order for it to be usable in internal workflows.
If uniform definitions are implemented well, it ensures consistency and equity in who gets to be called a prospect, what constitutes a major gift or what donor engagement looks like. Once this is fine-tuned, you can accomplish a healthier balance between the need for efficiency and the need to create consistent meaning with your data that is just and fair.
Goal Setting
The goal-setting agenda for data governance and DEI also shows promise for a natural connection between projects. While data governance protocols are generally applied to minimize risk and increase efficiency, those protocols are usually tied to organizational aspirations.
For example, if an organization wants to increase the value of their prospect data for business decisions, any data governance protocols around wealth screenings, the integration of external datasets such as Open Data and the incorporation of prospect feedback should reflect what will be accomplished with that data. This signals the data points are integral to the organization’s success, and not just rule-setting for rule-setting’s sake.
However, in creating a data governance framework to accomplish organizational goals, we should ask who the goal is serving and whether it diminishes other organizational aspirations. Additionally, when these goals are translated through the efficiency lens of data governance, it is likely that the goals that do not fit this mold will be given less priority.
This can create an environment where the focus on efficiency will influence organizational aspirations. For example, leadership could have a goal to disaggregate all constituent demographic information (i.e., labeling all demographics within your organization’s database), but are currently locked into a CRM that is limited in providing that ability. This leads to an environment where rules around disaggregation are confined to utilizing the CRM efficiently, diminishing the impact of disaggregating data among your constituents.
In situations like these, we end up prioritizing organizational goals that fit onto a model of efficiency rather than transforming the status quo. This dynamic empties DEI initiatives before they can be truly impactful. When confronted with the complexity of DEI initiatives, we might be tempted to create data protocols around DEI principles that fit our current structures, forgetting that substantive DEI initiatives require a transformational shift in how we understand our work. In this case, data governance rules strip the weight that DEI initiatives need to transform institutional practices.
That said, utilizing data governance rules to accomplish an organizational goal and ensuring the weight of DEI initiatives stay intact does not need to be a zero-sum game. For example, if we prioritize effectiveness over efficiency when thinking about organizational goals, we can ensure our data governance rules align with what it means to truly implement our DEI aspirations. Defining the effects of DEI aspirations once they are implemented forces us to find an efficient way of seeing it through. In this case, efficiency is still a priority but is no longer the driving force of aspiring toward an organizational goal.
Where Data Analytics Fits In
Data analytics is a key juncture point for data governance and DEI. In some organizations, data governance rules are created to support analytic workflows; in others, a data governance framework is created to establish data analytics within the organization. In all cases, if the output from data analysis defines the narrative of your organizational data, data governance could be thought of as providing the available corpus of words to that story. A data analytic pipeline is only as productive as the data governance rules make it.
This is where we can see the generative possibilities of data governance. Rather than a set of rules and protocols that constrain action, particularly in service of data analytics, data governance rules and protocols can be thought of as a catalyst for organizational action.
Just like DEI initiatives, data analytic workflows need room to be iterated upon for substantive impact. This makes data analytics a natural juncture point for DEI initiatives — both can only flourish if your organization’s data governance framework is agile and responsive to the organization’s needs. However, the dynamic between data governance, data analytics and DEI likely needs its own book to fully unpack the different nuances involved.
If your organization does not have an iterative environment for your data analytic workflows, your DEI initiatives are also likely to suffer. If no culture of iteration exists within your organization, it likely stems from your data governance rules. These will serve as the best starting point for adjustment to ensure your data analytic workflows and DEI initiatives are paired well.
Final Thoughts
It is my belief that the focus on efficiency in data governance protocols is not the best path forward to incorporate DEI initiatives into organizational settings. Rather, establishing a consistent meaning of your data in all organizational contexts, creating equitable and inclusive goals within your data governance frameworks, and ensuring iterative approaches to your analytic pipelines are all more suitable ways to make data governance work for DEI initiatives.
The implementation of each project is relatively new to our field, and we have a golden opportunity to guide how they interact with each other. While this article should not be seen as a how-to guide on exactly how to accomplish that, I hope it helps start the conversation and provides insights on the possible path forward.

Steve Grimes
Director of Development Analytics and Strategy, Jazz at Lincoln Center
Steve Grimes is the director of development analytics and strategy at Jazz at Lincoln Center. He oversees all reporting and analysis needs for senior leadership to help drive decision-making around fundraising activities. Previously, he was the prospect research analyst at the ACLU, working with the principal gifts team in the national office. Steve began his development career in 2006 as a prospect researcher at St. John’s University. He received a bachelor’s degree in psychology from SUNY at Old Westbury, a master’s degree in sociology from St. John’s University and another master’s degree in media studies from CUNY at Brooklyn College. In addition, he completed doctoral courses at Rutgers University before deciding to focus solely on his development career.